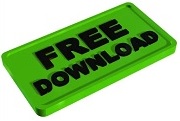

Those will allow us to derive practical optimisation algorithms from SGP. We discuss discretisation strategies for SGP. If the learning rate is decreasing to zero and additional assumptions hold, we will prove that SGP converges weakly to the Dirac measure concentrated in the global optimum. In this case, SGP is exponentially ergodic. We study the long-time behaviour of SGP: We give assumptions under which SGP with constant learning rate has a unique stationary measure and converges to this measure in the Wasserstein distance at exponential rate. We show that SGP is a sensible continuum limit of SGD and discuss SGP from a biological viewpoint: a model of the same type is used to model growth and phenotypes of clonal populations living in randomly fluctuating environments. We construct the stochastic gradient process (SGP), a continuous-time representation of SGD. More precisely, the contributions of this work are: In this work, we study the stochastic processes generated by the stochastic gradient descent (SGD) algorithm. However, understanding these properties seems crucial for the construction of efficient stochastic optimisation methods.

Stochastic properties of these processes have been hardly studied in the literature so far see Benaïm ( 1999), Dieuleveut et al. ( 2017).ĭue to the randomness in the updates, the sequence of iterates of a stochastic optimisation algorithm forms a stochastic process rather than a deterministic sequence. This is highly relevant for target functions in, e.g., deep learning, since those are often non-convex see Choromanska et al. Aside from a higher efficiency, this randomness can have a second effect: The perturbation introduced by subsampling can allow to escape local extrema and saddle points. In subsampling the aforementioned small fraction of the data set is picked randomly in every iteration. The stochasticity of the algorithms is typically induced by subsampling. Stochastic optimisation algorithms that only consider a small fraction of the data set in each step have shown to cope well with this issue in practice see, e.g., Bottou ( 2012), Chambolle et al. This leads to an immense computational cost.
#STOCHASTIC GRADIENT DESCENT FULL#
Those methods require evaluations of the loss function with respect to the full big data set in each iteration. Classical algorithms being gradient descent or the (Gauss–)Newton method see Nocedal and Wright ( 2006). Solving this problem with classical optimsation algorithms is usually infeasible. The training is usually phrased as an optimisation problem. The training of models with big data sets is a crucial task in modern machine learning and artificial intelligence. We conclude after a discussion of discretisation strategies for the stochastic gradient process and numerical experiments. In this case, the process converges weakly to the point mass concentrated in the global minimum of the full target function indicating consistency of the method. Then we study the case, where the learning rate goes to zero sufficiently slowly and the single target functions are strongly convex. We give conditions under which the stochastic gradient process with constant learning rate is exponentially ergodic in the Wasserstein sense. After introducing it, we study theoretical properties of the stochastic gradient process: We show that it converges weakly to the gradient flow with respect to the full target function, as the learning rate approaches zero. Processes of this type are, for instance, used to model clonal populations in fluctuating environments. The dynamical system-a gradient flow-represents the gradient descent part, the process on the finite state space represents the random subsampling. The stochastic gradient process is a dynamical system that is coupled with a continuous-time Markov process living on a finite state space. In this work, we introduce the stochastic gradient process as a continuous-time representation of stochastic gradient descent. Stochastic gradient descent is an optimisation method that combines classical gradient descent with random subsampling within the target functional.
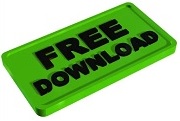